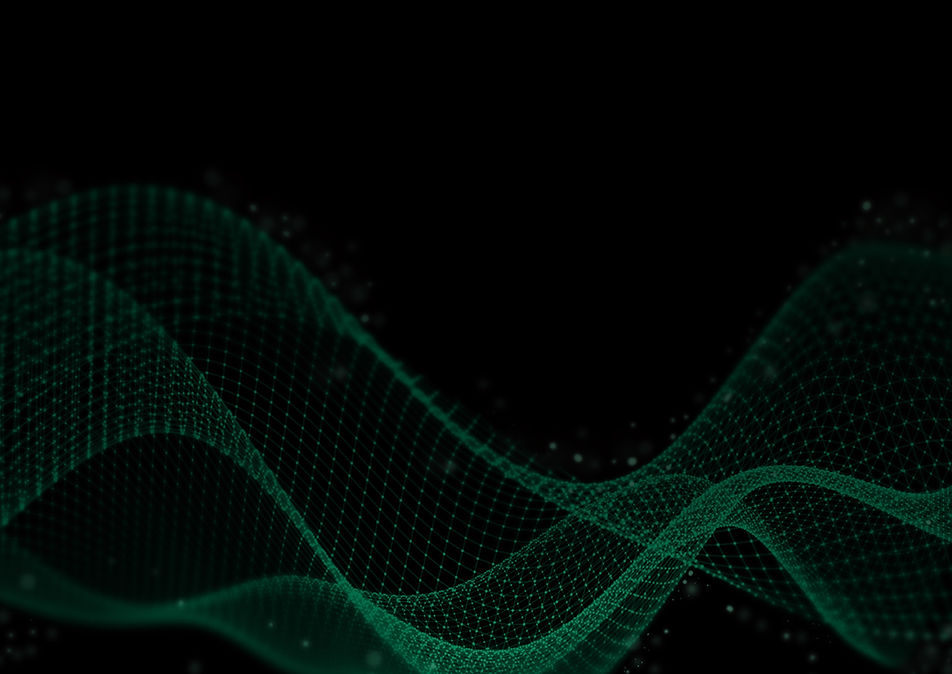
The Problem
Machine learning (ML) pipelines require large amounts of data to build, train and deploy ML models. Developing efficient ML pipelines is key to successfully leveraging AI.

Low algorithmic transparency is a major problem.
The better the quality of the data/datasets used to feed the machine learning pipelines, the better the outcomes.
But, there are several considerations, regarding the data used, which are of increasing importance, including:
-
What are the sources of the data used?
-
Is all of the data used correctly/legitimately?
-
Is the data trustworthy?
-
Is data stored securely?
-
How has the data been “prepared” for the ML pipeline?
Regulatory Compliance
The UK, EU and US are working on regulatory frameworks and potential legislation for companies operating ML/AI processes. These frameworks will include the already existing regulations covering data protection and digital systems, etc.
Companies with ML/AI operations are required to prove that their operations are fully compliant with the relevant and appropriate regulations and complex patchwork of legal requirements. This is difficult, and costly, to do across numerous regulations, legislations and jurisdictions.
Legal Challenges
The decisions made/actions taken by ML driven processes are open to challenge by any person or company affected by those decisions.
In cases where those challenges become legal it will be necessary for the operators to provide accurate and incontestable information regarding all data sources, data, datasets, processing parameters, models/algorithms used in the processes being challenged, potentially from the start of the process and up to the moment the decision being challenged was made.
There have already been several such high-profile legal challenges.
While the EU is legislating to implement a rules-based approach to AI governance, the UK is proposing a ‘contextual, sector-based regulatory framework’, anchored in its existing, diffuse network of regulators and laws.
The UK approach, set out in the white paper, “Establishing a pro-innovation approach to AI regulation”, rests on two main elements: AI principles that existing regulators will be asked to implement, and a set of new ‘central functions’ to support this work.
In addition to these elements, the Data Protection and Digital Information Bill currently under consideration by the UK Parliament is likely to impact significantly on the governance of AI in the UK, as will the £100 million Foundation Model Taskforce and AI Safety Summit convened by the UK Government.
A recent UK government survey found that over a fifth of organisations who are currently non-users of AI, but plan to introduce it, flagged uncertainty about regulation and legal responsibility as a barrier to adoption (“The roadmap to an effective AI assurance ecosystem).
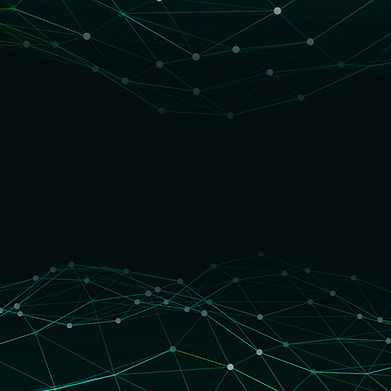